Within the domain of cancer research, survival analysis is used to understand which factors influence the chance of survival of patients diagnosed with cancer, e.g. the patient’s fitness, the method of treatment, or hospital of diagnosis. Traditionally, this type of analysis requires that all of these factors are part of a single database in order to study their effects. However, when these features are collected by various institutions and are considered privacy-sensitive it becomes significantly harder to include them in your study. This can have a significant consequence on research by limiting it to the data any particular researcher has access to. As such, TNO and IKNL are collaborating to develop methods for survival analysis on distributed data sources in a privacy-preserving manner.
Vertically-partitioned survival analysis
When various entities hold different pieces of information on the same group of people, we often refer to this scenario as the vertical partitioning of data. An analysis on vertically-partitioned data, that is without centralizing the data, requires more complex techniques. Therefore, we use the latest developments in cryptography, in particular, secure multi-party computation (MPC).
MPC is an umbrella term for cryptographic techniques that allows several different entities to jointly perform analysis on data without sharing their actual data. IKNL and TNO are collaborating to develop solutions using these technologies to enable privacy-preserving training of survival analysis models (e.g. Kaplan-Meier estimator, Log Rank Test, Cox regression, etc.)
We aim to deliver an open-source library for secure survival analysis on vertically-partitioned data. Once development has finished, the intention is to collaborate with other organisations to perform a joint analysis and hopefully get a better understanding of which factors might influence our chances of surviving from cancer.
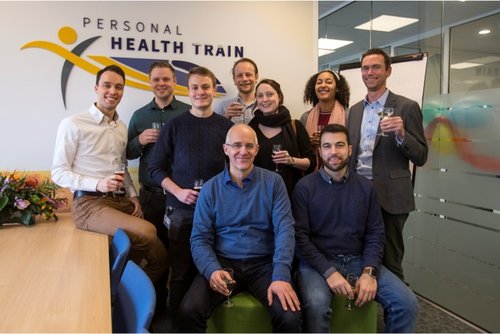
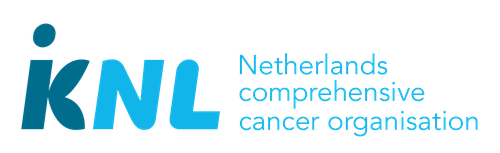
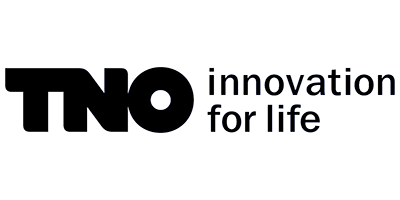